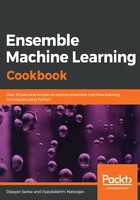
上QQ阅读APP看书,第一时间看更新
Introduction to sampling
Sampling techniques can be broadly classified into non-probability sampling techniques and probability sampling techniques. Non-probability sampling techniques are based on the judgement of the user, whereas in probability sampling, the observations are selected by chance.
Probability sampling most often includes simple random sampling (SRS), stratified sampling, and systematic sampling:
- SRS: In SRS, each observation in the population has an equal probability of being chosen for the sample.
- Stratified sampling: In stratified sampling, the population data is divided into separate groups, called strata. A probability sample is then drawn from each group.
- Systematic sampling: In this method, a sample is drawn from the population by choosing observations at regular intervals.
If the sample is too small or too large, it may lead to incorrect findings. For this reason, it's important that we've got the right sample size. A well-designed sample can help identify the biasing factors that can skew the accuracy and reliability of the expected outcome.
Errors might be introduced to our samples for a variety of reasons. An error might occur due to random sampling, for example, which is known as a sampling error, or because the method of drawing observations causes the samples to be skewed, which is known as sample bias.