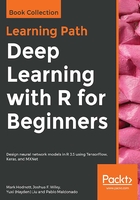
Activation functions
The activation function determines the mapping between input and a hidden layer. It defines the functional form for how a neuron gets activated. For example, a linear activation function could be defined as: f(x) = x, in which case the value for the neuron would be the raw input, x. A linear activation function is shown in the top panel of Figure 4.2. Linear activation functions are rarely used because in practice deep learning models would find it difficult to learn non-linear functional forms using linear activation functions. In previous chapters, we used the hyperbolic tangent as an activation function, namely f(x) = tanh(x). Hyperbolic tangent can work well in some cases, but a potential limitation is that at either low or high values, it saturates, as shown in the middle panel of the figure 4.2.
Perhaps the most popular activation function currently, and a good first choice (Nair, V., and Hinton, G. E. (2010)), is known as a rectifier. There are different kinds of rectifiers, but the most common is defined by the f(x) = max(0, x) function, which is known as relu. The relu activation is flat below zero and linear above zero; an example is shown in Figure 4.2.
The final type of activation function we will discuss is maxout (Goodfellow, Warde-Farley, Mirza, Courville, and Bengio (2013)). A maxout unit takes the maximum value of its input, although as usual, this is after weighting so it is not the case that the input variable with the highest value will always win. Maxout activation functions seem to work particularly well with dropout.
The relu activation is the most commonly-used activation function and it is the default option for the deep learning models in the rest of this book. The following graphs for some of the activation functions we have discussed:
